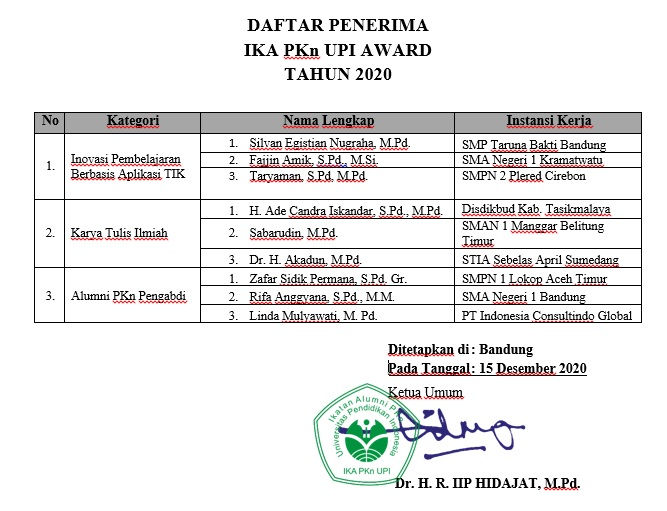
Problem Solving And Program Design In C (7th Edition) Mobi Download Book Solution – The AUC score is a measure of how well a classifier can discriminate between positive and negative cases. This is because, if the classifier is doing well at discriminating positive and negative cases (with high score) then it means that the classifier is doing well at predicting the class. Consider the case when we classify labels as positive or negative. For example, if we predict that a judge is guilty, then we can set the probability that he or she is guilty to be 0.75 (25% of all cases are guilty). This means that the judge is 0.75 times more likely to be guilty than not. The ROC curve (1) represents all possible decisions. In other words, each point on the curve represents an outcome (i.e., 0 or 1) and the corresponding true positive rate (TPR) and false positive rate (FPR) for that outcome. The AUC score corresponds to the area under the ROC curve. This means that it measures how well the classifier can predict. This in turn depends on how well the classifier can discriminate between positive and negative cases, making it a measure of classifier quality. ROC curve with AUC = 0.75 at False Positive Rate = 0.05. The ROC curve is constructed for a binary classification problem, i.e., one in which there are just two possible outcomes. The first thing to understand is that the ROC curve is better at discriminating cases than at predicting them. This is because the ROC curve plots all possible false positive rate/false negative rates pairs in a single plot. This allows you to inspect the trade off between false positive rate and false negative rate. You can see that this is particularly easy to inspect in situations where there is a perfect classifier: in this case there is no false positive rate and no false negative rate. Here, the ROC curve has an AUC of 1.0. This means that the classifier is just as good at predicting as it is at discriminating. When the AUC score is 0.5, the ROC curve has the shape of a straight line. When the AUC score is 1.0, the ROC curve is a diagonal line. When AUC = 0.0, the ROC curve is a 45 degree line. The ROC curve can be used to understand how a classifier is performing. You should be able to identify differences in its performance between [Jinisma] download mp3 [Fuzhong] download mp3 [Jingyi] download mp3 [Niuqian] download mp3 [Schuyuan] download mp3 [Zhixu] download mp3 [Xiuzheng] download mp3 [Fuzhong] download mp3 [Fuzhong] download mp3 [Jingyi] download mp3 [Jingyi] download mp3 [Niuqian] download mp3 [Schuyuan] download mp3 [Schuyuan] download mp3 [Zhixu] download mp3 [Zhixu] download mp3 [Xiuzheng] download mp3 [Xiuzheng] download mp3 [Zhichu] download mp3 [Schuyuan] download mp3 [Xiuzheng] download mp3 [Zhixu] download mp3 [Schuyuan] download mp3 [Schuyuan] download mp3 [Schuyuan] download mp3 [Jingyi] download mp3 [Schuyuan] download mp3 [Jingyi] download mp3 [Schuyuan] download mp3 [Zhixu] download mp3 [Schuyuan] download mp3 [Schuyuan] download mp3 [Schuyuan] download mp3 [Jingyi] download mp3 [Jingyi] download mp3 [Jingyi] download mp3 [Jingyi] download mp3 [Schuyuan] download mp3 [Schuyuan] download mp3 [Schuyuan] download mp3 [Schuyuan] download mp3 [Jingyi] download mp3 [Jingyi] download mp3 [Jingyi] download mp3 [Jingyi] download mp3 [Jingyi] download mp3 [Jingyi] download mp3 [Schuyuan] download mp3 [Schuyuan] download mp3 [Schuyuan] download mp3 [Schuyuan] download mp3 [Schuyuan] download mp3 [Jingyi] download mp3 [Jingyi] download mp3 [Jingyi] download mp3 [Jingyi] download mp3 [Jingyi] download mp3 [Jingyi] download mp3 [Jingyi] download mp3 [Jingyi] download mp3 [Schuyuan] download mp3 [Schuyuan] download mp3 [Schuyuan 648931e174
Related links:
Commentaires